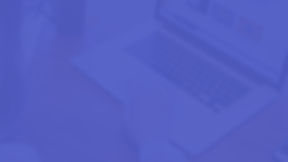
HUMANE
INTELLIGENCE
AI Product Readiness at Scale.
OUR
SERVICES
CONSULTING & STAFF AUGMENTATION
STRATEGY
PUBLIC POLICY
EDUCATION
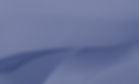
WHO
WE ARE
We are a non-profit founded and led by industry veterans and optimists, Dr. Rumman Chowdhury and Jutta Williams. Humane Intelligence supports AI model owners seeking product readiness review at scale.
​
We focus on safety, ethics, and subject-specific expertise. Our services are suited for any company creating consumer-facing AI products, but in particular generative AI products.
OUR
WORK
All humans can contribute to improved algorithms. Traditionally, companies that produce algorithms employ specialized teams that work behind closed doors to solve problems. Diverse issues with large-scale models are best solved by having more, diverse people contributing to solutions.
​
Humane Intelligence provides services and a platform for sourcing structured public opinion to improve product review. At our core, we are a 4th line of defense that provides a double-blind external assessment for how well your model performs. We help you identify the right questions to ask, acquire pools of experts to assess your product tailored for your use cases, and deliver useful reporting. We address the core problems of AI safety and ethics review at scale by utilizing a range of AI/ML models on the backend to ensure raw data quality and to parse actionable insights.
We offer multiple tiers of services:
-
Self-serve. Companies design and deploy their own product review, selecting their pricing and expert pool size. Companies receive raw output files, however HI ensures high signal ratio by utilizing our proprietary models and provides periodic data updates.
-
Co-creation. Companies co-create with HI. HI provides design input, suggested sample size and payouts. We provide periodic reporting and suggestions.
-
HI developed. HI fully designs and deploys the review with minimal specifications from our clients
​
All studies undergo an ethics review by our IRB and all participants are paid a fair wage for their time, including education and onboarding. By employing a blind or double-blind service, model developers can engage in feedback anonymously while eliminating the challenge of identifying cohorts and the hazard of collecting tester PII.
​
Examples:
-
Medical Mis-information: Identifying a pool of specialized medical experts to assess LLM outputs for misleading or incorrect recommendations.
-
High-risk industrial AI systems: Identifying industry field experts who can assess generative model designs for safety.
-
Testing Foundational LLM model for LGBTQ biases by conducting a double-blind feedback program for curated community member
The Problem
General-purpose AI tools, such as generative AI, have a potentially infinite range of misuse ranging from incorrect information (‘hallucinations’) to toxic and abusive content. These adverse outcomes can be risky for product trustworthiness, compliance and reputational risk.
​
Scaling red-teaming services with a high level of accuracy is an unsolved problem.
​
Companies making generative AI models do not have easy ways to tackle these problems at scale, first because of the massive potential for harm, and second, because of the sheer volume and scale of adverse events that are produced.
​
Companies creating plugins or specialized applications of commercial generative AI models need to further test and refine these models for readiness to market.
These companies share the following problems:
-
Identifying individuals for demographic-specific testing requires storing information about the individual that may violate privacy law
-
Sourcing and training individuals is difficult and time-consuming
-
Information received from at-scale crowdsourcing (ie RLHF) has a high noise to signal ratio
-
It is difficult to parse at-scale red team feedback in order to understand when risk assessment is complete
Scaling red-team services
Specialized Tools
Staffing & Training
OUR
CLIENTS
Our client list right now is private but we hope to share more as public announcements are available.
GET IN
TOUCH
We can't wait to hear from you! ​